Stay ahead of the curve with our Newsletter!
Join the Updated Course on Building with AI in 2025
〰️
Join the Updated Course on Building with AI in 2025 〰️
Learn how to build any software with AI
Join the 1st Batch of AI Software Builders
The Updated 1st batch of the world’s most enabling course on AI. Learn how to build anything with AI.
What is Generative AI?
What are LLMs and SLMs?
What is a RAG?
What are AI Agents?
Generative AI creates new content, like text or images, based on patterns in data. Large Language Models (LLMs) are a powerful form of this AI, generating human-like text, while Small Language Models (SLMs) focus on specialized tasks with less data. Retrieval-Augmented Generation (RAG) enhances these models by pulling in external information for more accurate results.
AI Agents use generative AI to autonomously perform tasks such as writing or research. Together, they represent cutting-edge advancements in automation and creativity.
Learn it all on generativeai.net.
“AI can not only boost our analytic and decision-making abilities but also heighten creativity.”
— Harvard Business Review
The Power of Generative AI
Generative AI is revolutionizing communication, work, and innovation.
With tools like ChatGPT, Gemini, and ClaudeAI reaching millions of users, its impact is undeniable.
Its widespread use on GitHub shows its transformative power. Already reshaping industries, its influence will only expand.
Let’s explore some key applications:
Text Generation
Text Generation involves using machine learning models to generate new text based on patterns learned from existing text data. The models used for text generation can be Markov Chains, Recurrent Neural Networks (RNNs), and more recently, Transformers, which have revolutionized the field due to their extended attention span. Text generation has numerous applications in the realm of natural language processing, chatbots, and content creation.
🪄 Application: ChatGPT, developed by OpenAI, is a successful platform that uses Text Generation to generate human-like responses in chat conversations.
Example of ChatGPT in use
Image Generation
Image Generation is a process of using deep learning algorithms such as VAEs, GANs, and more recently Stable Diffusion, to create new images that are visually similar to real-world images. Image Generation can be used for data augmentation to improve the performance of machine learning models, as well as in creating art, generating product images, and more.
🪄 Application: Very successful platforms such as MidJourney and DALL-E have become a popular choice for anyone seeking to generate realistic images through Image Generation techniques.
Image generated with MidJourney
Video and speech Generation
Video Generation involves deep learning methods such as GANs and Video Diffusion to generate new videos by predicting frames based on previous frames. Video Generation can be used in various fields, such as entertainment, sports analysis, and autonomous driving. Video Generation can be often seen in use with Speech Generation. The models used for speech generation can be powered by Transformers. Speech Generation can be used in text-to-speech conversion, virtual assistants, and voice cloning.
🪄 Application: Platforms such as DeepBrain and Synthesia utilize Video and Speech Generation to create realistic video content, that appears as if a human was speaking on camera.
Video fully generated (including the human avatar) with DeepBrain AI Studio. Prompt given: “How to prepare for a spaceship adventure as a cat”.
Data Augumentation
Data augumentation is a process of generating new training data by applying various image transformations such as flipping, cropping, rotating, and color jittering. The goal is to increase the diversity of training data and avoid overfitting, which can lead to better performance of machine learning models.
🪄 Application: Synthesis AI simplifies the process of building and optimizing machine learning models by providing a platform for creating AI models using automated machine learning techniques.
…And much more
Generative AI has a diverse range of applications that go beyond text, video, image, speech generation, and data augmentation. For instance, it can be used for music generation, game development, healthcare, and more. In healthcare, generative AI can help generate synthetic medical data to train machine learning models, develop new drug candidates, and design clinical trials. These are just some examples of the many possibilities for generative AI, and as the technology advances, we can expect to see more applications emerge.
It's an exciting time to dive into Generative AI! With this field in its early stages, those who gain the necessary skills and knowledge have the opportunity to shape its future. Get ready for an exciting journey!
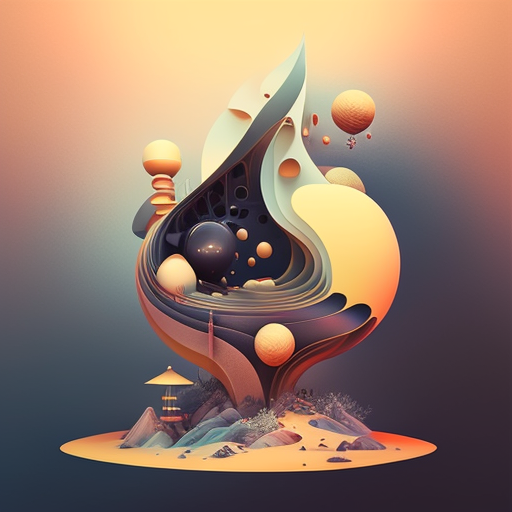
Want to learn more about Generative AI?
Get the Course
Join our comprehensive online course and learn the fundamentals of this cutting-edge topic area. Whether you're a beginner or an experienced professional, this course will equip you with the knowledge you need to succeed. Enroll now!